Identifying Road Crashes Before They Occur
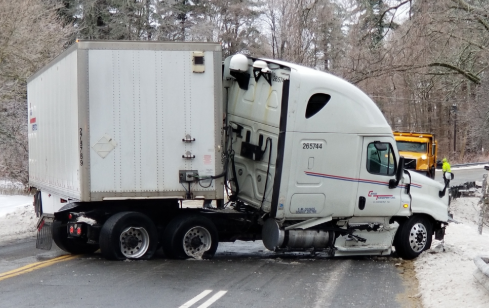
EROAD has created a new data algorithm that will enable transport authorities to move to a predictive program of risk management that can improve road safety
Driver behavior gathered from over 9.5 million trips and collected through EROAD technology was anonymized, aggregated then analyzed through an innovative algorithm that has resulted in a new way of identifying risk with unparalleled accuracy.
“We wanted to create a model that could predict where crashes were most likely to occur so that preventative measures could be implemented before those areas became known as high-crash risk areas” said EROAD’s Director of Analytics Gareth Robins. “When compared to traditional methods of risk analyses, our technology and new algorithm is an efficient and effective way of comparing multiple factors gathered from a range of vehicle types, giving unprecedented information on how our roads are being driven”.
The research was conducted using data from New Zealand and looked at fatigue, frustration and familiarity, then compared these factors across the five classes of New Zealand roads.
Fatigue looked at the distance from where the vehicle started its trip. Unsurprisingly, this found that roads leaving State Highways in provincial areas were identified as riskier than other roads, because of the longer travel time taken to reach them.
Frustration was measured by drivers’ propensity for speed changes >10 km/hr over the speed limit when there was a change in road curvature. This looked at drivers’ overspeed when moving from a corner to a segment of straight road or from a straight road segment to another segment of straight road.
For familiarity the research focused on harsh braking and speeding, as known precursors to a crash, then looked at where these occurred in relation to the final trip destination. There were higher occurrences of harsh braking towards the end of trips, with 50% occurring within 8.6km of a vehicle’s destination.
These three factors, frustration, fatigue and familiarity, were then combined using a further algorithm that produced a level of risk for a defined segment of roading. This showed the level of risk of an incident occurring, known as the Dynamic Risk, and is shown in Fig 1.
Dynamic Risk was developed by Gareth Robins, Director of Analytics at EROAD and Dr Salvador Hernandez, Assistant Professor Civil & Construction Engineering at Oregon State University.
EROAD is known for its technology
solutions that manage vehicle fleets, support regulatory compliance and improve
driver safety. The data collected is anonymized and aggregated for research use
ensuring those who use the roads are influencing the design, management and
funding of future transport networks.
Category: Featured, General Update, Management, Safety, Tech Talk